In recent years, generative AI has emerged as a transformative force in business, offering unprecedented capabilities in content creation, data analysis, and decision-making processes. However, as enterprises rush to adopt this powerful technology, they face a complex landscape of security challenges that demand careful consideration and strategic planning.
Introduction to Generative AI in Enterprises
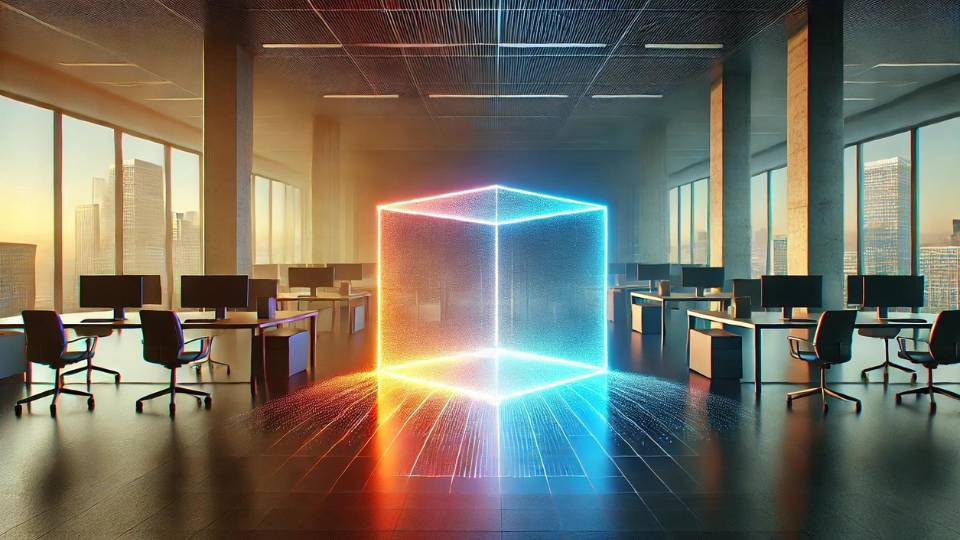
Generative AI refers to artificial intelligence systems that create new content, such as text, images, or code, based on patterns learned from existing data. In enterprise settings, these systems are revolutionizing operations across various departments, from customer service to product development. The adoption of generative AI has seen a significant upswing, with industries ranging from finance to healthcare leveraging its potential to streamline processes and drive innovation.
According to recent studies, the global generative AI market is projected to grow at a compound annual growth rate (CAGR) of over 30% in the coming years. This rapid adoption is driven by the technology’s ability to enhance productivity, reduce costs, and create new business opportunities. For instance, financial institutions use generative AI to improve fraud detection and personalize customer experiences, while manufacturing companies employ it to optimize supply chain management and product design.
Security Concerns in Generative AI
While the benefits of generative AI are clear, its implementation brings forth a host of security concerns that organizations must address to ensure safe and responsible use.
Data Privacy and Protection
One of the primary concerns surrounding generative AI is the risk of sensitive data exposure. These AI models often require vast amounts of training and operation data, including confidential business information, personal customer data, or proprietary intellectual property. The Cisco 2024 Data Privacy Benchmark Study reveals that 63% of organizations have set limitations on data input into generative AI systems to mitigate these risks.
The potential for data breaches or unauthorized access to the AI models themselves poses significant threats to an organization’s privacy and security posture. Enterprises must implement robust data protection strategies, including encryption, access controls, and data anonymization techniques, to safeguard sensitive information in AI systems.
AI Model Safety
The safety and integrity of AI models themselves are crucial concerns. Issues related to model robustness, bias, and transparency can lead to unreliable outputs or compromised decision-making processes. There’s also the risk of model tampering, where malicious actors could alter the AI’s behavior or extract sensitive information embedded in the model.
Organizations need to prioritize AI model discovery, risk assessment, and security measures to address these concerns. This includes regular audits of AI models, implementing secure AI frameworks, and establishing protocols for model updates and maintenance.
Misuse and Cyber Attacks
The advanced capabilities of generative AI also present opportunities for misuse in cyber attacks. Malicious actors could leverage these systems to generate convincing phishing emails, create deepfakes for social engineering attacks, or automate malware creation. The ability of AI to mimic human communication patterns makes it a powerful tool for sophisticated social engineering campaigns.
According to Splunk’s “State of Security 2024 Report,” there’s been a notable increase in AI-powered cyber attacks, with many organizations reporting encounters with AI-generated phishing attempts and deepfake-based fraud.
Challenges in Managing Generative AI
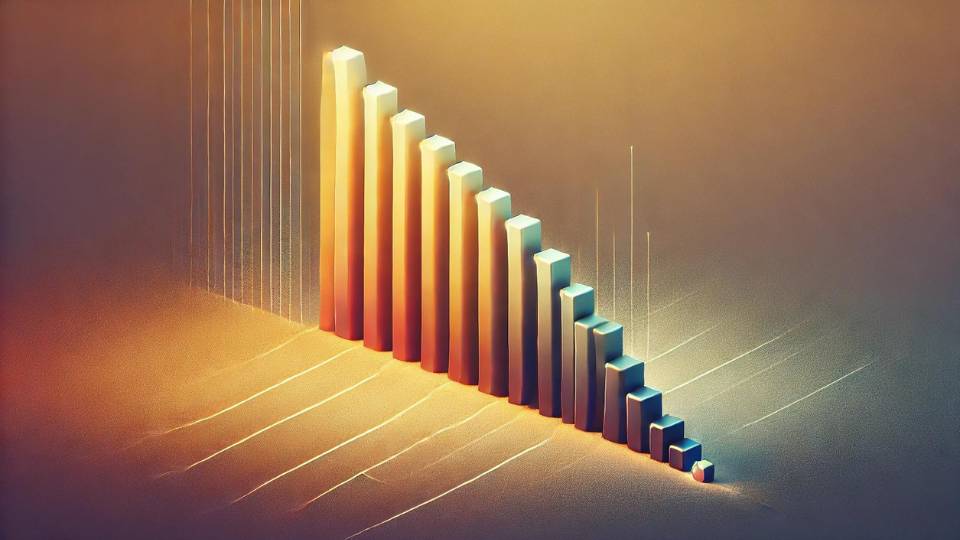
The implementation and management of generative AI systems present several challenges organizations must navigate to ensure secure and practical use.
Lack of Governance Frameworks
As generative AI rapidly evolves, many organizations need robust governance frameworks to manage these systems effectively. Deloitte’s “State of Generative AI in the Enterprise 2024” report indicates that 90% of organizations believe new techniques are needed to handle AI data and risk.
Establishing comprehensive governance policies is crucial for addressing security concerns, ensuring regulatory compliance, and maintaining ethical standards in AI use. This includes defining clear roles and responsibilities, setting guidelines for AI development and deployment, and implementing monitoring and auditing processes.
Technical Talent Shortage
The complexity of generative AI systems requires specialized skills to manage and secure them effectively. However, many organizations need more professionals with expertise in AI security, model development, and risk management.
This talent gap can lead to vulnerabilities in AI implementations and hinder an organization’s ability to respond to emerging security threats. Investing in training programs and partnering with AI security experts can help address this challenge.
Trust and Transparency Issues
Building trust in AI systems is crucial for their successful implementation and adoption. However, there’s often a gap between consumer expectations for data transparency and how businesses currently manage AI applications. The Cisco study highlights that 84% of consumers want more transparency about how their data is used in AI applications.
To address this, organizations should prioritize explainable AI techniques, implement clear data usage policies, and consider obtaining external privacy certifications to build stakeholder trust.
Case Studies of Reluctance and Issues Post-Adoption
The security concerns surrounding generative AI have led some organizations to approach its adoption cautiously, while others have faced significant challenges after implementation.
Reluctance to Adopt
According to Splunk’s report, over 27% of organizations have banned generative AI applications to avoid potential risks. For example, several major financial institutions have imposed strict limitations on using generative AI tools like ChatGPT due to concerns about data privacy and regulatory compliance.
Some law firms have prohibited using generative AI for document drafting in the legal sector, citing risks of confidentiality breaches and potential inaccuracies in AI-generated content.
Problems Encountered
Several high-profile cases have highlighted the risks associated with generative AI adoption:
- A significant tech company faced backlash when it was revealed that its customer service AI had access to sensitive user data, leading to privacy concerns and potential regulatory violations.
- A healthcare provider experienced a data breach when their AI-powered diagnostic system was compromised, exposing patient records and medical histories.
- A financial services firm suspended its AI-driven trading algorithm after it made a series of erroneous trades, resulting in significant economic losses.
These incidents underscore the need for comprehensive security measures and risk assessment strategies when implementing generative AI systems.
Mitigation Strategies and Best Practices
To address the security concerns associated with generative AI, organizations should consider implementing the following strategies:
Data Command Centers
Establishing centralized data command centers can help organizations monitor and manage AI systems more effectively. These centers can oversee data flows, track AI model performance, and respond quickly to potential security incidents.
Enhanced Data Controls
Implementing comprehensive data inventory audits, classification systems, and access controls is crucial for protecting sensitive information used in AI systems. Organizations should conduct regular data privacy impact assessments and implement data minimization techniques to reduce exposure risks.
AI Safety Measures
Adopting robust AI model safety practices is essential. This includes:
- Regular risk assessments of AI models
- Developing secure AI frameworks to prevent model tampering and data exfiltration
- Implementing model versioning and rollback capabilities
- Conducting adversarial testing to identify potential vulnerabilities
Future Outlook and Recommendations
As generative AI evolves, the security landscape will undoubtedly become more complex. Organizations must stay ahead of emerging threats and adapt their security strategies accordingly.
Evolving Security Landscape
Future trends in generative AI security are likely to include:
- Advanced AI-powered threat detection and response systems
- Increased focus on AI model interpretability and explainability
- Development of industry-specific AI security standards and certifications
- Integration of blockchain technology for enhanced AI data integrity and traceability
Recommendations for Enterprises
To balance the benefits of generative AI with robust security measures, organizations should:
- Develop a comprehensive AI governance framework that addresses security, ethics, and compliance.
- Invest in continuous monitoring and auditing of AI systems to detect and respond to potential security threats.
- Prioritize staff training on AI security best practices and potential risks.
- Collaborate with industry partners and regulatory bodies to establish and adhere to AI security standards.
- Implement a zero-trust security model for AI systems, assuming no user or system is inherently trustworthy.
- Regularly update and patch AI models and supporting infrastructure to address emerging vulnerabilities.
Conclusion
Generative AI presents tremendous opportunities for enterprises to innovate and streamline operations. However, the associated security risks must be addressed. Organizations can harness the power of generative AI while maintaining a strong security posture by understanding these concerns, implementing robust security measures, and staying informed about evolving threats.
As technology advances, the importance of addressing security concerns in generative AI will only grow. Enterprises prioritizing AI security and governance will be better positioned to leverage the full potential of this transformative technology while protecting their assets, reputation, and stakeholders.